Methodology
BiT is a a cutting-edge model or blur interpolation, constructed using Multi-scale Residual Swin Transformer Blocks (MS-RSTBs). To enhance the performance of BiT for blur interpolation, we have incorporated two temporal strategies, namely Dual-end Temporal Supervision (DTS) and Temporally Symmetric Ensembling (TSE). DTS involves the use of temporal supervision at both ends of the exposure time, while TSE involves ensembling the features obtained from forward and backward directions of the same time point. These strategies lead to a significant improvement in the performance of BiT for blur interpolation.
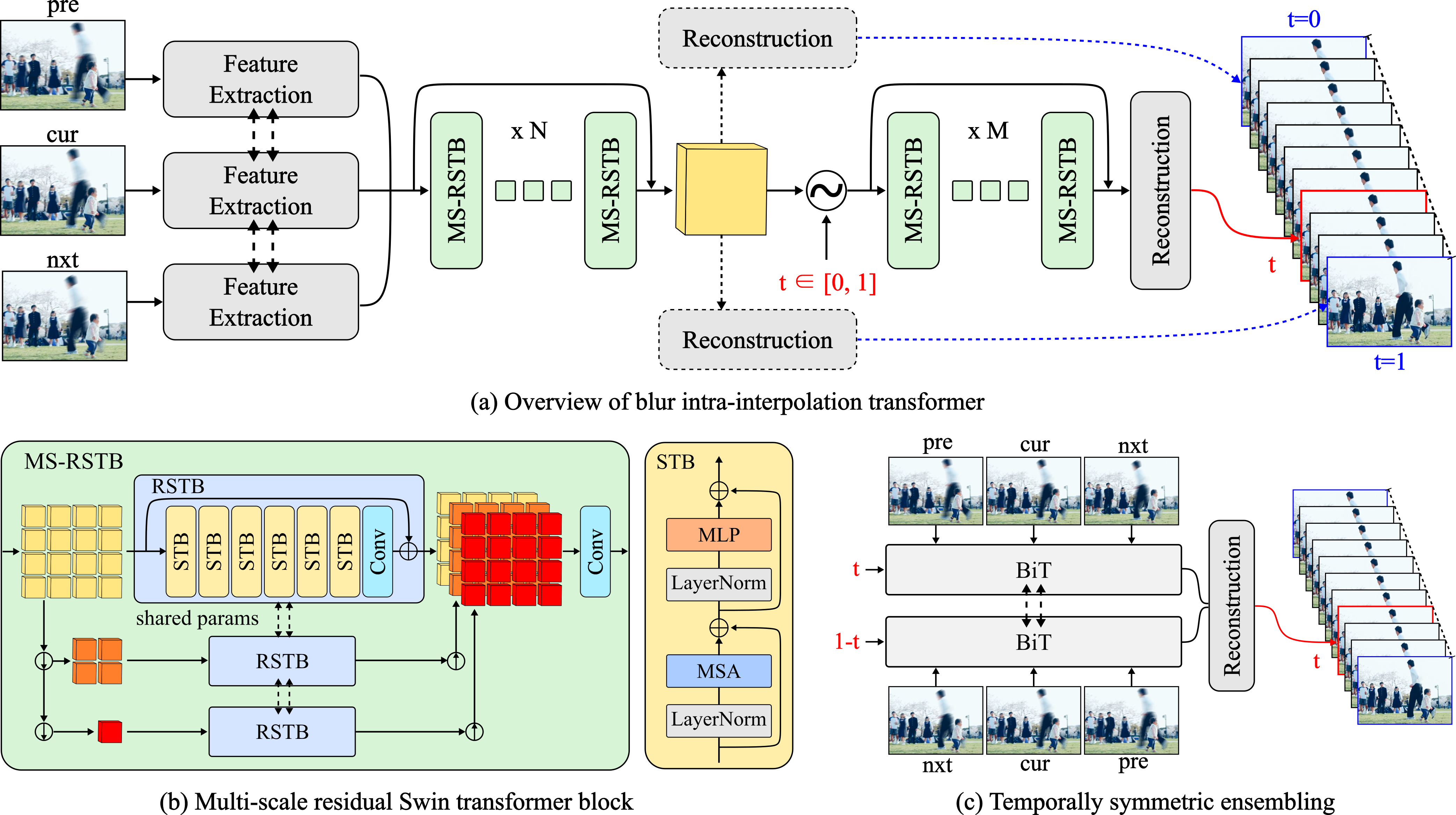